Team 1: Observational Error Related Negativity for Trust Evaluation in Human Swarm Interaction
Joseph P. Distefano (University of Buffalo)
This groundbreaking study marks the inaugural exploration of Observation Error Related Negativity (oERN) as a pivotal indicator of human trust within the paradigm of human-swarm teaming, while simultaneously delving into the nuanced impact of individual differences, distinguishing between experts and novices. In this institutional Review Board (IRB) approved experiment, human operators physiological information is recorded while they take a supervisory control role to interact with multiple swarms of robotic agents that are either compliant or non-compliant. The analysis of event-related potentials during non-compliant actions revealed distinct oERN and error positivity (Pe) components localized within the frontal cortex.
Extended AbstractShort VideoPoster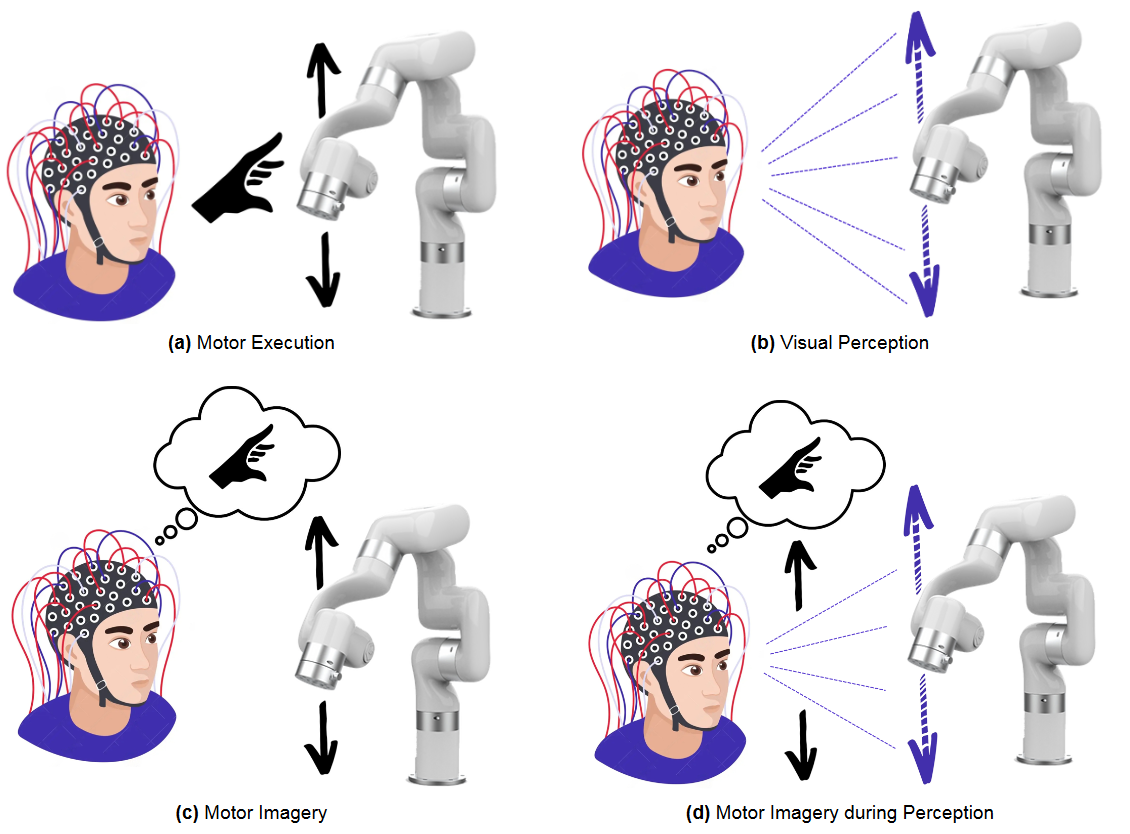
Team 2: Novel Intuitive BCI Paradigms for Decoding Manipulation Intention - Imagined Interaction with Robots
Matthys Du Toit (University of Bath)
Human-robot interfaces lack intuitive designs, especially BCIs relying on single-body part activation for motor imagery. This research proposes a novel approach: decoding manipulation intent directly from imagined interaction with robotic arms. EEG signals were recorded from 10 subjects performing motor execution, visual perception, motor imagery, and imagery during perception while interacting with a 6-DoF robotic arm. State-of-the-art classification models achieved average accuracies of 89% (motor execution), 94.9% (visual perception), 73.2% (motor imagery), and highest motor imagery classification of 83.2%, demonstrating feasibility of decoding manipulation intent from imagined interaction. The research invites more intuitive BCI designs through improved human-robot interface paradigms.
Short VideoPoster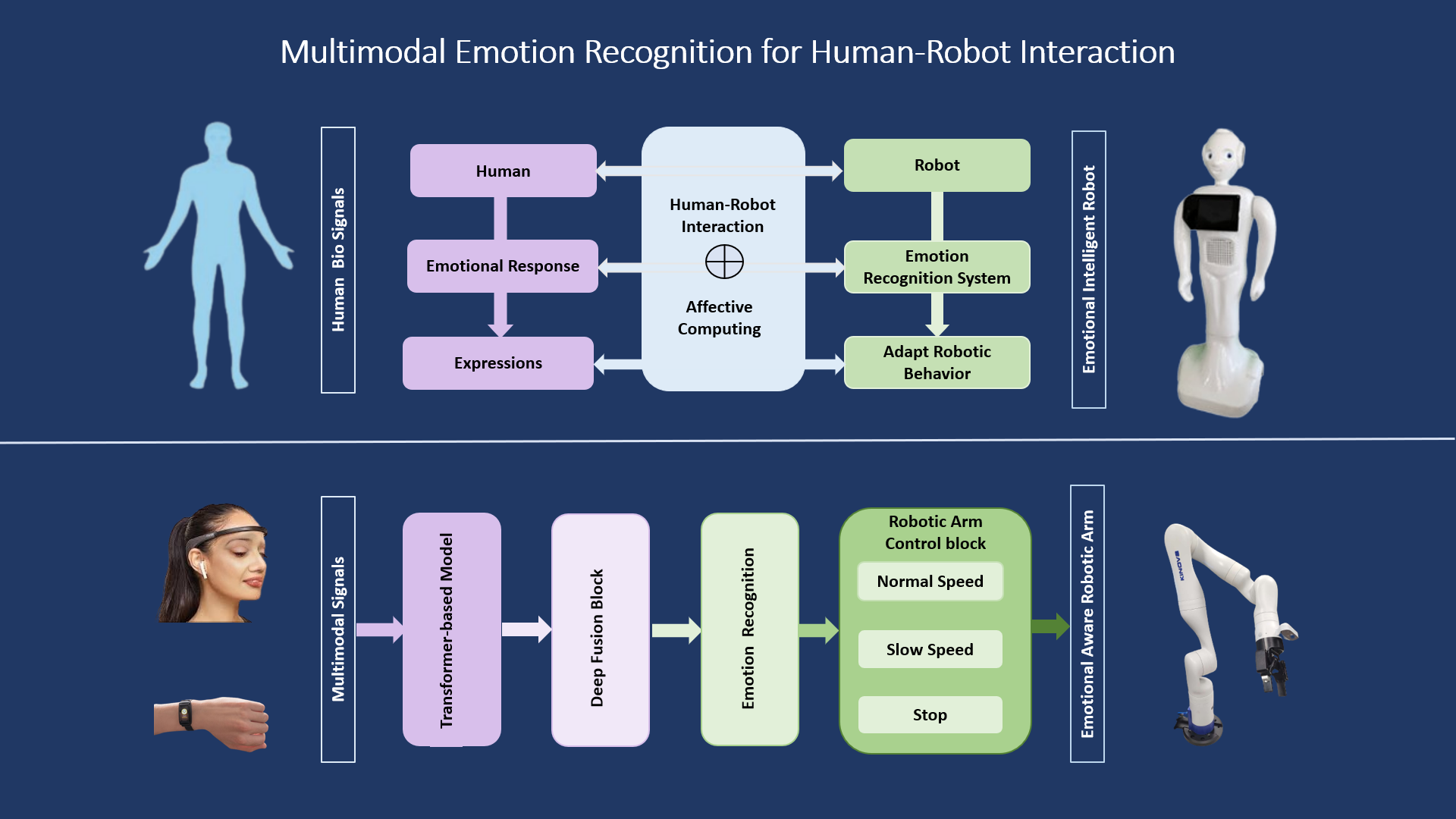
Team 3: Multimodal Emotion Recognition for Human-Robot Interaction
Farshad Safavi (University of Maryland, Baltimore County)
Our project is a multimodal emotion recognition system to enhance human-robot interaction by controlling a robotic arm through detected emotions, using facial expressions and EEG signals. Our prototype adjusts the robotic arm's speed based on emotions detected from facial expressions and EEG signals. Two experiments demonstrate our approach: one shows the arm's response to facial cues—it speeds up when detecting happiness and slows down for negative emotions like angry face. The other video illustrates control via EEG, adjusting speed based on the user's relaxation level. Our goal is to integrate emotion recognition into robotic applications, developing emotionally aware robots.
Intro SlidesShort VideoPoster
Team 4: Learning Hand Gestures using Synergies in a Humanoid Robot
Parthan Olikkal (University of Maryland, Baltimore County)
Hand gestures, integral to human communication, hold potential for optimizing human-robot collaboration. Researchers have explored replicating human hand control through synergies. This work proposes a novel method: extracting kinematic synergies from hand gestures via a single RGB camera. Real-time gestures are captured through MediaPipe and converted to joint velocities. Applying dimensionality reduction yields kinematic synergies, which can be used to reconstruct gestures. Applied to the humanoid robot Mitra, results demonstrate efficient gesture control with minimal synergies. This approach surpasses contemporary methods, offering promise for near-natural human-robot collaboration. Its implications extend to robotics and prosthetics, enhancing interaction and functionality.
Intro SlidesShort VideoPoster.jpg)
Team 5: A Wearable, Multi-Channel, Parameter-Adjustable Functional Electrical Stimulation System for Controlling Individual Finger Movements
Zeyu Cai (University of Bath)
As the survival rate of patients with stroke and spinal cord injuries rises, movement dysfunction in patients after surgery has become a concern. Among them, hand dysfunction seriously impairs patients' quality of life and self-care ability. Recent, many studies have demonstrated that functional electrical stimulation (FES) in the rehabilitation of upper limb motor function. At the same time, compared to traditional treatment methods, functional electrical stimulation is more effective. However, existing FES studies for the hand placed the electrodes in the forearm, which does not allow full control of individual movements of single fingers. In this study, an electrode glove was designed to place the electrodes on the hand, which can achieve this goal. Furthermore, existing FES systems are large in size, the FES system developed in this study is more lightweight and can be made wearable. In summary, this study aimed to develop a novel, wearable functional electrical stimulation system for the hand, which can adjust the stimulation parameters and, with an electrode glove, can control the individual movements of single fingers providing a personalized rehabilitation approach.
Extended AbstractIntro SlidesPoster
Team 6: Enhancing MI-BCI Training with Human-Robot Interaction Through Competitive Music-Based Games
Alessio Palatella (University of Padova)
Motor Imagery Brain-machine Interfaces (MI-BMIs) interpret users' motor imagination to control devices, bypassing traditional output channels like muscles. However, MI-BMIs' proficiency demands significant time and effort, especially for novices. To address this, we propose a novel MI-BMI training method using Human-Robot Interaction via rhythmic, music-based video games and NAO robots. Our experimental setup involves a rhythm game connected to a real NAO robot via a BMI. EEG signals are processed using a CNN-based decoder. Despite data limitations, our approach demonstrates promising control capabilities, highlighting the potential of combining MI-BMIs with robotics for intuitive human-robot interaction and enhanced user experience.
Extended AbstractIntro SlidesShort VideoPoster
Team 7: Enhancing Synergy - The Transformative Power of AR Feedback in Human-Robot Collaboration
Akhil Ajikumar (Northeastern University)
Through this paper, we introduce a novel Augmented Reality system to enable intuitive and effective communication between humans and collaborative robots in a shared workspace.By using multimodal interaction data like gaze, speech, and hand gestures, which is captured through a head-mounted AR device, we explore the impact created by the system in improving task efficiency, communication clarity, and user trust in the robot. We validated this using an experiment, based on a gearbox assembly task, and it showed a significant preference among users for gaze and speech modalities, it further revealed a notable improvement in task completion time, reduced errors, and increased trust among users. These findings show the potential of AR systems to enhance the experience of human-robot teamwork by providing immersive, real-time feedback, and intuitive communication interfaces.
Extended AbstractPoster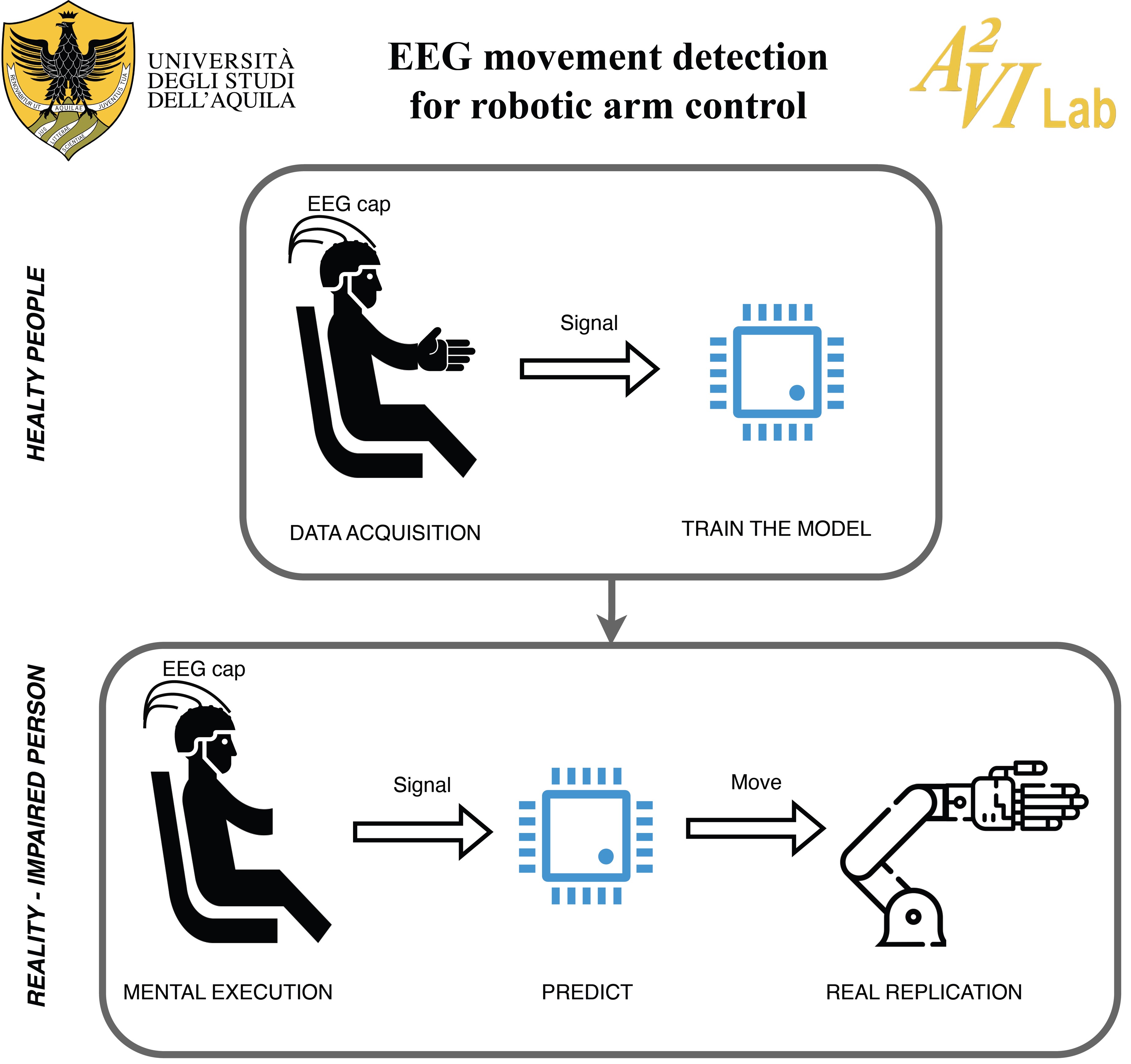
Team 8: EEG Movement Detection for Robotic Arm Control
Daniele Lozzi (University of L'Aquila)
This research introduces a novel approach to the construction of an online BCI dedicated to the classification of motor execution, which importantly considers both active movements and essential resting phases to determine when a person is inactive. Then, it explore the best Deep Learning architecture suitable for motor execution classification of EEG signals. This architecture will be useful for control an external robotic arm for people with severe motor disabilities.
Extended AbstractPoster
Team 9: EEG and HRV Based Emotion Estimation Robot for Elderly Interaction
Yuri Nakagawa (Shibaura Institute of Technology)
The increasing demand for emotional care robots in nursing homes aims to enhance the Quality of Life for the elderly by estimating their emotions and providing mental support. Due to the limited physical state of elderly, traditional methods of emotion estimation pose challenges; thus, we explore physiological signals as a viable alternative. This study introduces an innovative emotion estimation method based on Electroencephalogram and Heart Rate Variability, implemented in a care robot. We detail an experiment where this robot interacted with three elderly individuals in a nursing home setting. The observed physiological changes during these interactions suggest that the elderly participants experienced positive emotions.
Intro SlidesPoster